ICTP-East African Institute for Fundamental Research
KIST2 Building CST
Nyarugenge Campus
University of Rwanda
Kigali, Rwanda
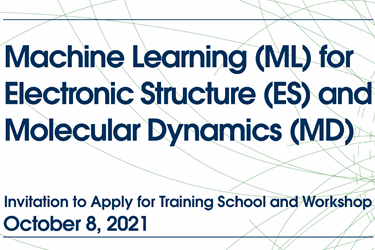
Machine Learning for Electronic Structure and Molecular Dynamics
Applications will be received till end of October, 2021.
In the study of known materials and the prediction of the properties of new ones, electronic structure (ES) methods as well as atomistic simulations such as molecular dynamics (MD) have proved to be formidable tools and have facilitated the development of new materials. However, currently, ES and MD methods are limited by the size of the system such that only “small” sizes and “short” simulation times are practically possible for a given accuracy. Moreover, when a lot of data are generated by these methods, it may be hard to directly detect useful patterns that can help in the development of new materials. Finally, there are some problems where our understanding is incomplete and we do not even know how to apply ES and/or MD to tackle them (such as the study of non-conventional superconductivity). Machine learning (ML) and Artificial Intelligence (AI) offer systematic approaches to address all these problems, broadening the scope of traditional ES and MD simulations.
Application of ML in ES and MD is an area in which young researchers can work with relatively modest computational resources for the development of new materials and for providing deeper understanding of material behavior. ML offers great opportunities to young students and researchers in Africa to contribute in a massive way to scientific and technological development. Unfortunately, many of the young students and researchers in Africa are hardly exposed (or not exposed at all) to ML in ES and MD. We envision that increased collaboration between researchers in Africa and those in the rest of the world is vital in order to reduce this knowledge/skill gap and have more people all over the world engaged in scientific and technological development for the world benefit. To partially fill this gap, we are organizing a combined school and workshop.
A six-month training school in Machine Learning and its application to Electronic Structure and Molecular Dynamics is organized for researchers in Africa. It will take place online from October 2021 to April 2022 and will culminate in a workshop in Kigali, Rwanda in the period 16 – 20 May, 2022. The six-month training will require attendance of online classes one to three times a week on average, with a total time of 2—7 hours each week, The broad topics for the six-month training include:
- Introduction to Python programming
- Basics of Machine Learning (ML)
- Working with Materials Databases (Materials Project, NOMAD, AFLOW) and OPTIMADE
- Combining ML with Materials Databases for Materials Discovery
- Machine Learning for Force Field development
- Machine Learning in Electronic Structure
At the end of the six-month training, the top 20 participants will be selected to attend the final workshop in Kigali, Rwanda 16-20 May, 2022, while the other participants will join online. The workshop in Kigali will also feature research talks from top scientists in the field and poster presentations.
Who should apply?
Lecturers in Africa who carry out research in electronic structure and/or atomistic/molecular modeling and are interested in the application of Machine Learning in their research. These include those who use electronic structure methods and/or sampling methods such as Monte Carlo and Molecular Dynamics in different fields: Condensed Matter Physics, Materials Science, Computational/Quantum Chemistry, Computational Drug Design and others areas. Postdoctoral fellows and advanced post-graduate students at the masters or doctoral levels working in Africa can also apply. Women are particularly encouraged to apply.
How to apply:
To apply, fill out the Google form at https://forms.gle/62f387Nt62jGZSb9A
In addition, please send a copy of your CV to MLESMD@eaifr.org.
First deadline for application is 14/October/2021 and the training will start on Monday/18/October/2021.
Applications will be received till end of October, 2021.
For further information contact: MLESMD@eaifr.org.
Download this information in poster format.
Organizers:
Omololu Akin-Ojo (ICTP-EAIFR, Rwanda)
Ali Hassanali (ICTP, Trieste Italy)
Alessandro Laio (SISSA, Italy)
Steve Ndengue (ICTP-EAIFR, Rwanda)
Gian-Marco Rignanese (UCLouvain, Belgium)
Matthias Scheffler (FHI-MPG Berlin, Germany)
Lecturers/Speakers:
Joerg Behler (Georg-August Univ, Germany)
Michele Ceriotti (EPFL, Switzerland)
Bingqing Cheng (IST, Austria)
Gabor Csanyi (Univ. Cambridge, UK)
Stefano Curtarolo (Duke Univ., USA)
Pierre-Paul De Breuck (UCLouvain, Belgium)
Stefano de Gironcoli (SISSA, Italy)
Marco Esters (Duke Univ., USA)
Emine Kucukbenli (Boston Univ., USA)
Alessandro Laio (SISSA, Italy)
Michele Parrinello (ETH Zurich, Switzerland)
Gian-Marco Rignanese (UCLouvain, Belgium)
Matthias Scheffler (FHI-MPG Berlin, Germany)
Yusuf Shaidu (UC Berkeley, USA)
Omololu Akin-Ojo (ICTP-EAIFR, Rwanda)
Current Sponsors:
ICTP-EAIFR/UR, Psi-k Network